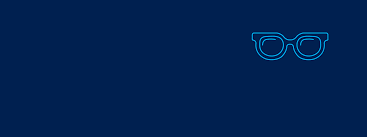
It is Almost 2020🙄🙄. Do you know how many pictures you took, Do you know how much digital images we have, Do you know what is a state of the image today. It is impossible to predict. Images have become ubiquitous in production and consumption. It is the right time to know about computer vision. Let's start 👀👀.
"Every picture tells us a story"
What is computer vision
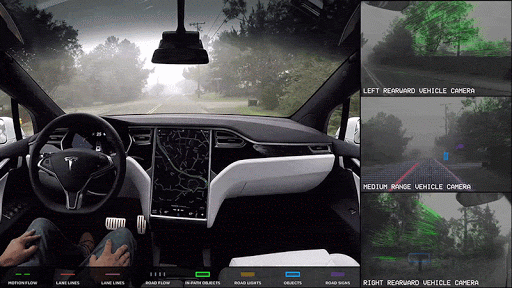
Computer vision is a field of artificial intelligence that trains computers to interpret and understand the visual world. The goal of computer vision is to write computer programs that can interpret images.
Can we compare computer vision with human vision 🤷♀️🤷♀️?
If you ask me this I will say it is madness. Human vision is far away from computer vision
The human eye is capable of processing visual information far more quickly than any computer. While the human brain can instantly recognize objects or human faces, a computer has to add together the information pixel by pixel
You may hear about image processing. In image processing, an image is "processed", that is, transformations are applied to an input image and an output image is returned. Computer vision uses image processing algorithms to solve some of its tasks.
How is computer vision-related with deep learning🤔🤔
The field of computer vision is shifting from statistical methods to deep neural network methods. There are still many challenging problems to solve in computer vision. Nevertheless, deep learning methods are achieving state-of-the-art results on some specific problems.
Before 20 years back we are lack of computational power, good storage, and data. deep neural networks are failed to achieve the state-of-the-art. At that time computer vision is entirely dependent on the statistical methods. Know we are having good computational power through GPU and TPU's.deep neural networks achieved many things that's why computer vision shifted from statistical methods to the deep neural network.
Where is computer vision ⁇
since we are in the world of visuals there are many applications of computer vision some of the applications are given below.
optical character recognization:
optimal character recognization (OCR) is a system that provides a full alphanumeric recognization of printed or handwritten characters.
Google lens is using the OCR system to detect the characters on the image and show information available on the web related to that text.

OCR systems are using in banks, post office, the government is using to detect the number on number plates on vehicles, and there many more places where OCR is using.
object detection:
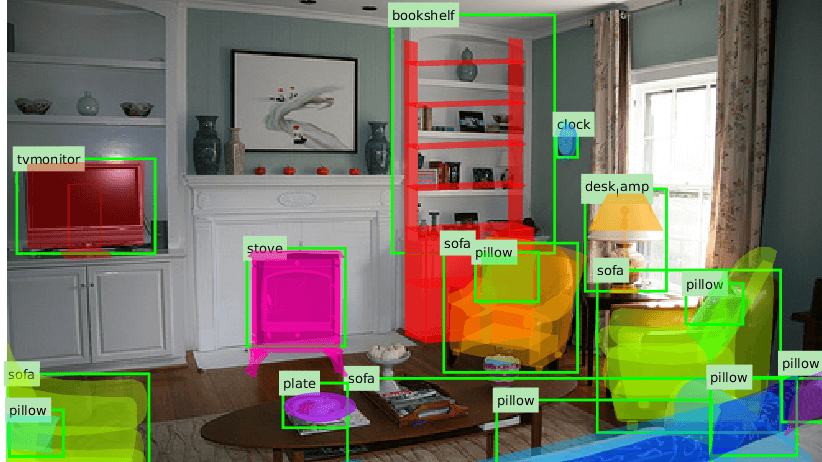
Object detection is very familiar to us as we are humans we can detect objects very easily compared to the computer. it is a tough task for them. Over the year there is lots of research going on in this area. In recent years we got good results. object detection is one of the mentionable achievements achieved by deep learning.
Google Glass uses object detection to detect the objects seen through our eyes and gives information about those objects.
motion capture and 3D modeling:
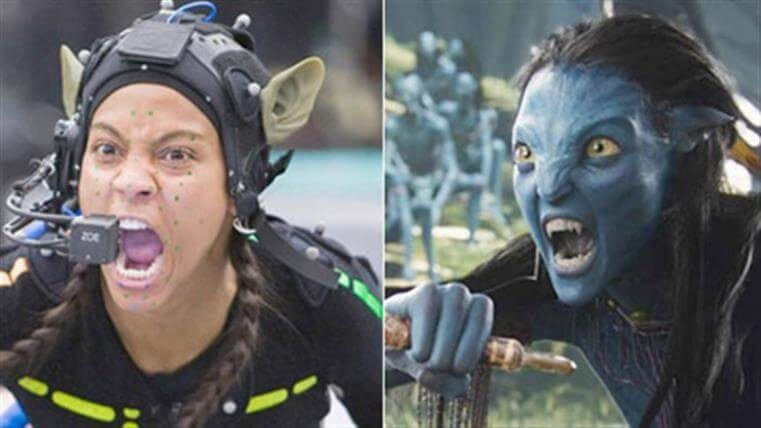
computer vision is known using in many movies for motion capturing. using computer vision the motion in the picture is captured and captured motion is used in various ways like duplicating character, to create an animated character as above.
self-driving cars:
it is my favorite application of computer vision. A self-driving car (sometimes called an autonomous car or driverless car) is a vehicle that uses a combination of sensors, cameras, radar and artificial intelligence (AI) to travel between destinations without a human operator. To qualify as fully autonomous, a vehicle must be able to navigate without human intervention to a predetermined destination over roads that have not been adapted for its use.
Companies developing and/or testing autonomous cars include Audi, BMW, Ford, Google, General Motors, Tesla, Volkswagen, and Volvo. Google's test involved a fleet of self-driving cars -- including Toyota Prii and an Audi TT -- navigating over 140,000 miles of California streets and highways.
Jobs outlook and salary:
According to the U.S. Bureau of Labor Statistics (BLS), computer and information research scientists, which include computer vision engineers, could expect a 19% rise in jobs between 2016 and 2026, which is much faster growth than the average American occupation during that period. Yet, this is a small field, meaning that within this 10-year period there will only be 5,400 new job openings for these scientists.
In 2018, the median salary for computer and information research scientists was found to be $118,370 per year, according to the BLS. The lowest 10% were said to earn $69,230 per year while the highest 10% made $183,820 per year. For points of comparison, Payscale.com noted that computer vision engineers earned $91,856 as an average salary in 2019, while Glassdoor.com published an average salary for those engineers of $87,001 that same year.
Computer vision engineers often have skills working with linear algebra math libraries and other similar computer vision libraries. They will need to have various software skills in the areas of database management, development environment, and component or object-oriented software. Analytical and critical-thinking skills are important because these engineers work on complex problems and must be able to analyze results for making accurate conclusions. Logical thinking, clear reasoning, and being detailed-oriented are also very important.
Tools for computer vision:

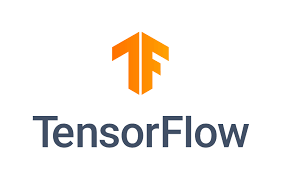
OpenCV:
OpenCV is the most popular library for computer vision. It is multi-platform and easy to use.
OpenCV provides various algorithms for the processing of images and videos. We can use OpenCV in python and c++.
Applied when creating image processing apps and basically built for prototyping and research purposes. Its code is quite succinct, easy to read, and debug. It also tackles a problem of the errors: proposes some ways to speed up code before being executed.
Tensorflow:
Google’s open-source framework for deep learning, has some great tools to perform image processing/classification — it is something similar to API graph tensor. Moreover, Python API can be used to perform face and expression detection. Tensorflow also allows performing computer vision of tremendous magnitudes.
CUDA:
NVIDIA’s platform for parallel computing that is easy to program and quite efficient and fast. Leveraging the power of GPUs it delivers great performance. Its toolkit includes the NVIDIA Performance Primitives library comprised of a set of image, signal, and video processing functions.
Thank you for reading this blog😀😀.
The next of blogs will concentrate on computer vision topics🧐🧐.
Comments